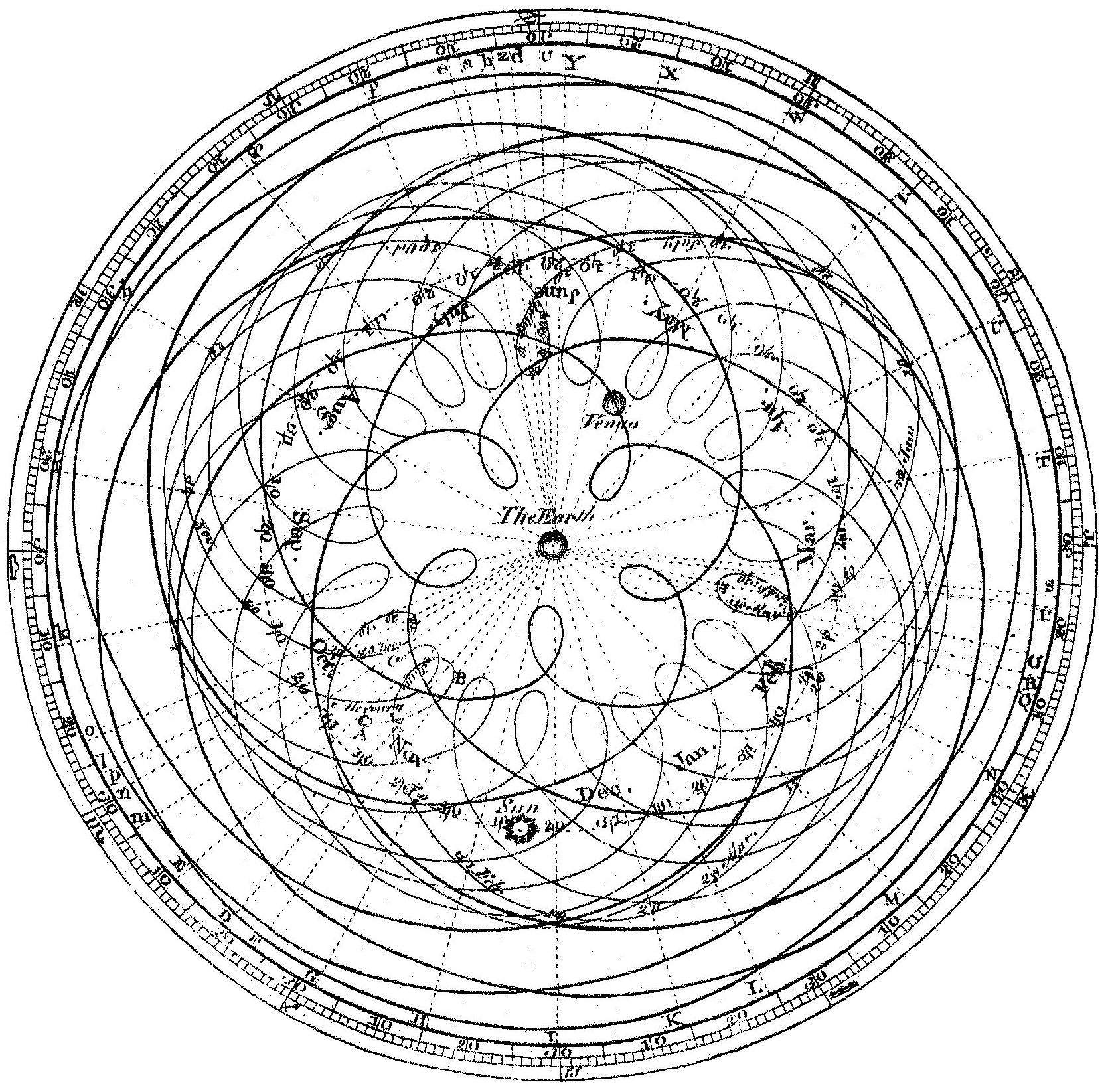
Contesting the distinction of machine learning models being either tools or models of neural computation
In our observations of the discussions within the computational neuroscience community, there has been a recurring debate: are machine learning models merely tools, or do they serve as models of neural computation? Common opinions in this field range from views like, “Yes, a DNN model is a black box, but if it accurately predicts outcomes, it’s a valuable tool, regardless of our understanding of its underlying mechanisms,” to “The structure of an ML model precludes it from being a model of reality. Even if the computations are correct, differences in implementation (as per Marr’s levels) imply that these should not be considered representations of actual brain processes.” Another perspective is, “An ANN that is sufficiently constrained, learns from natural stimuli, and adheres to biologically plausible update rules can be an excellent model of neural computation, especially if it predicts responses and generalizes effectively.”
Generally, these statements are not mutually exclusive, and each has its own merits. However, in this brief note, we wish to suggest that the question need not be posed as an either/or scenario: a model or a tool?
If a tool proves to be highly effective, then it undoubtedly represents some form of a model (given it generalises well). Therefore, the crucial next step is to decipher its functioning. This doesn’t necessarily mean that the methods an ML model uses to make accurate predictions mirror the brain’s processes. However, it does imply that the model has developed a mechanism worth exploring. We propose that this could greatly benefit computational neuroscience. If each model known to be an effective tool is scrutinised to understand its workings, we can then compare these mechanisms with corresponding brain activity. Only after such comparative analyses can we conclude whether the ML procedure has yielded something that might be considered a model of reality.
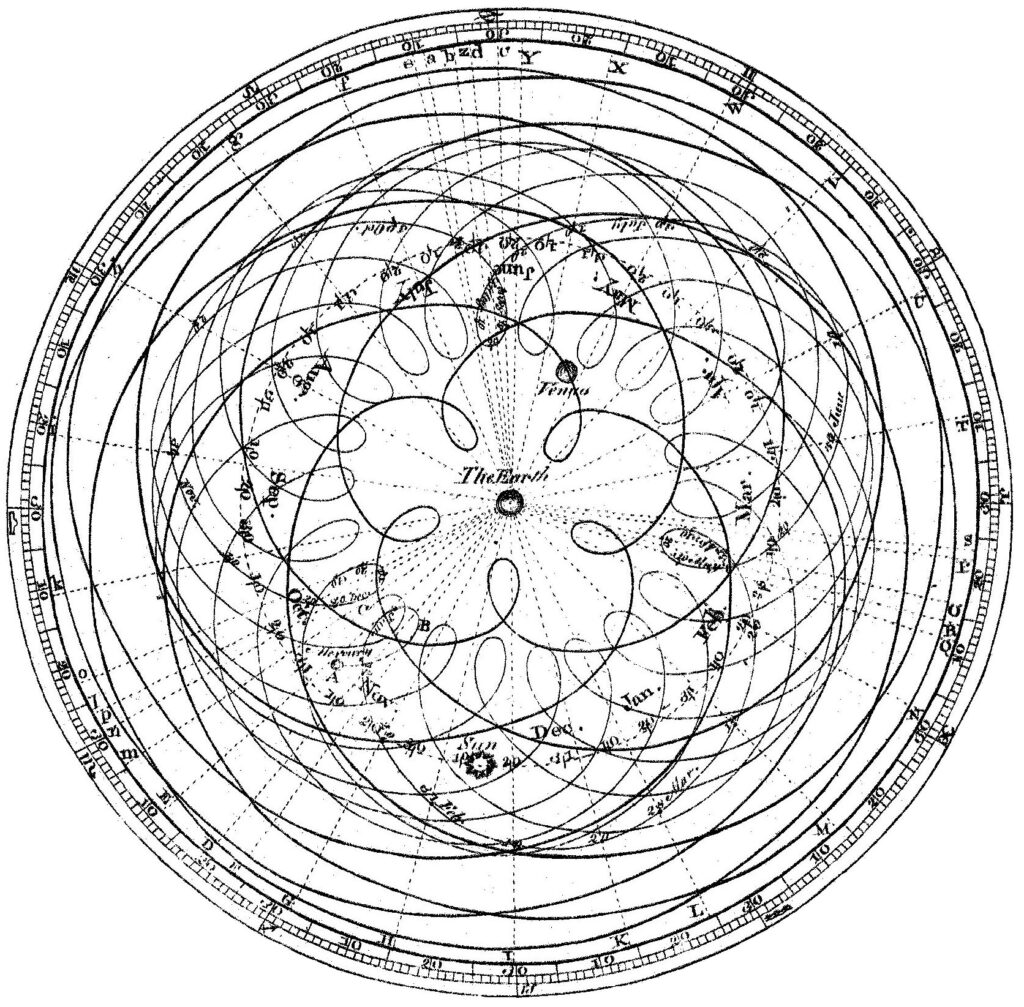
No comments yet.